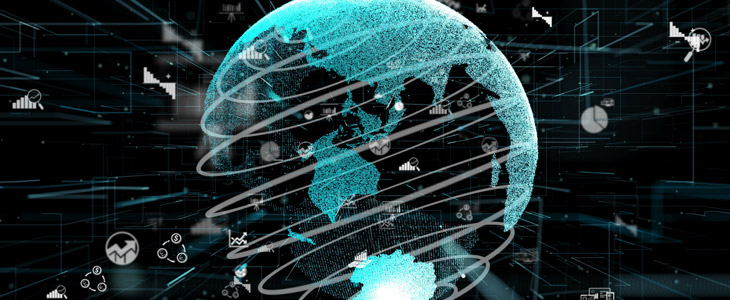
Countries have long fought financial crime by relying on financial institutions (FIs) to identify and report suspicious transactions. This system is largely ineffective because it rarely provides useful real-time feedback—a reality that is giving way to a more collaborative approach. This challenge is starting to be recognized now, and as a result there has been more collaboration.
Knowing how bad actors are trying to subvert the financial system is critical in stopping them. To maximize the value of information sharing, FIs must be able to share data among themselves and government agencies effectively.
Challenge: Blind Men and the Financial Crime Elephant
In the current system, when an entity is flagged as “suspicious” within an institution, the investigation and decision making is based on the available information within that institution, such as historical activity, client profile and some external information (e.g., media scan and high-risk list). Entity information available to one institution may represent a very small understanding of overall entity activity rather than a holistic view. Institutions can also reach out to each other to share information. However, that process relies on an investigator and requires manual information gathering and sharing by all the involved institutions. This makes the current information sharing model highly inefficient and expensive.
Solution: Next-Gen Collaboration Framework Using Graphs
Innovative technology like graphs―which succinctly express complex money movement patterns, detect interconnected relationships, and identify hubs and spokes of activity―can be a game-changer to future information sharing and a collaborative framework. A graph can provide the intuitive ability to present information as nodes or vertices like client attributes, external parties and accounts. In addition, it clarifies relationships or links between nodes, such as client to account and transactions between parties.
- Institution-explicit graph: Institutions can start by setting up their own graph model, which is essentially all their internal data such as clients, accounts, involved external parties, products and services, client profiles and historical cases. Internal systems such as onboarding platforms and core banking should regularly feed graph data models to keep it current. All the institutions that are part of the information sharing and collaboration platform should maintain their own institution-specific graph.
- Collective global graph: Based on an already agreed upon privacy-preserving model, all involved institutions can share a slice of their individual graph in a joint collective global graph. While this may contain limited information, it should contain the “key” information needed to understand the overall financial crime risk by other institutions. An externally available sanctions list, a high-risk list and other public-private data (beneficiary ownership) should also be included. Information available as a shared global graph can be automatically available for quick access.
- Areas to leverage shared graphs: Information from other institutions can be leveraged at various processes of the financial crime program, such as the following:
- Client onboarding and know your customer (KYC): The cost to KYC while onboarding a new client by individual institutions is massive. In addition, despite requiring the same information, all institutions repeat the same processes over and over. Therefore, institutions can leverage if the same client has been validated for identity verification and sanctions checks. Most importantly, gathering and verifying ultimate beneficial ownership is one of the most expensive processes, so it could be shared and enriched between FIs thereby leveraging enhanced client monitoring and investigating.
- Activity monitoring and detection: After the client onboarding is complete, institutions are required to monitor client activity for any suspicious money laundering or terrorist financing. Most frequent and emerging risk typologies should be shared among institutions. This will allow other institutions to prepare for emerging risk trends if unknown to them. In addition, “flagged” risky entities can be shared with other institutions.
- Investigation: Shared graphs provide quick access to information for an entity under investigation. These entities can be external parties for one institution and customers to others. An institution that has these “external parties” as customers can provide much enriched information to other institutions. In addition, institutions can collaborate on “joint” investigations, which can make the overall investigation very efficient and inexpensive.
- Regulatory reporting: Once a decision has been made to file a regulatory report, an institution should include information gathered from other institutions as part of the case narrative to provide enhanced information to regulators. Lastly, in the case of “shared investigations,” institutions should be able to file a “Joint Regulatory Report,” thereby making processing the regulatory reporting inexpensive overall.
Graph matching can provide a holistic view of all the matched entities by various attributes, such as name, address or email. This unifies data in real-time to create a single entity view across the enterprise. Entity resolution can be applied to resolve different entities based on shared addresses, phone numbers, tax IDs or names—key indicators to identify a drug ring network. In many cases, criminals use aliases or pseudonyms, which can be resolved by bringing internal and external data together for a consistent entity definition. Specifically, this is useful when criminals are using different products and services to “layer” within various institutions. At various stages of the process, institutions should be able to leverage from a shared global graph that includes both internal and external data for entity recognition and resolution.
New Considerations
This new model of information sharing and collaboration would require a great amount of collaboration between FIs, regulatory agencies and law enforcement. Below are some key items to consider:
- Agreement on shared information: The start of the whole platform is a mutual agreement on which information would be available to other peer institutions. Most importantly, there should be a technology platform to ensure the institution’s specific privacy policies.
- Tailored vs. global risk profile: Every institution’s risk profile is different; therefore, regardless of collaboration, institutions should tailor the program to their specific risk profile and appetite.
- Similar demographic: Institutions with shared demographic profiles could leverage the most from this collaborative model. For example, Indian banks, Canadian banks, mid-west American banks, Australian banks and so forth.
- Collaboration with regulators: For full leverage of such a platform, institutions should work with local regulators to derive the approach for shared investigations and a Joint Regulatory Report
Conclusion: Collective Intelligence and Learning
For the past years, governments and the financial sector have been teaming up to create information-sharing partnerships that facilitate a two-way dialogue on suspected financial crimes. When the two parties can share information on specific cases or on the types of potential criminal activity they are seeing, the industry can more effectively screen for suspicious activity.1
FIs can leverage powerful graph analytics to connect the dots between internal and external data, providing a holistic representation of networks that uncover hidden patterns. Investigators can click through entities and their connections—represented as nodes on the graph model—to analyze networks and suspicious activities. Artificial intelligence can also be leveraged to enhance human expertise through recommendations and next-best actions while helping analysts gain situational awareness and learn institutional best practices. Once detected by a machine learning model and designated by the investigator as a true positive, previously detected organized drug trafficking cases can be leveraged to make recommendations for new evidence in a graph. This way, institutions can ensure collective learning.
Garima Chaudhary, head of financial crime & compliance management solution consulting – Americas, Oracle, Toronto, Canada, garima.chaudhary@oracle.com
- Dylan Tokar, “Partnerships to Fight Financial Crime Gain Momentum,” The Wall Street Journal , August 18, 2020, https://www-wsj-com.cdn.ampproject.org/c/s/www.wsj.com/amp/articles/partnerships-to-fight-financial-crime-gain-momentum-11597743001